
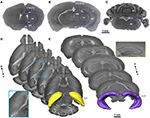
Targeted in situ sequencing (ISS) using padlock probes and localized rolling circle amplification provides submicron localization of RNA species in a highly multiplexed fashion in cells and entire tissue sections, and recent advancements in automation have led to higher detection efficiency and shorter protocol times. All approaches come with benefits and drawbacks, such as spatial resolution, depth of sequencing, accuracy, and throughput. The other approach is the parallel profiling of large numbers of mRNAs using barcodes decoded directly in the tissue sample. Generally described, there are two approaches to preserve spatial information: One approach is to either imprint a spatial reference on a grid or to carefully record spatial location prior to collection and sequencing of single-cell RNA. Several methods for measuring gene expression while preserving spatial information have been developed over the past years. We hypothesize that tissue and cell type can be defined by gene expression and that gene expression thus should define spatial compartments. Some regions, such as the pyramidal cell layer of the hippocampus in the brain, can be well defined based on morphology, whereas other regions are more difficult to identify as the morphological variations are small when cellular labeling is limited to nuclear staining or a finite set of fluorescent markers.
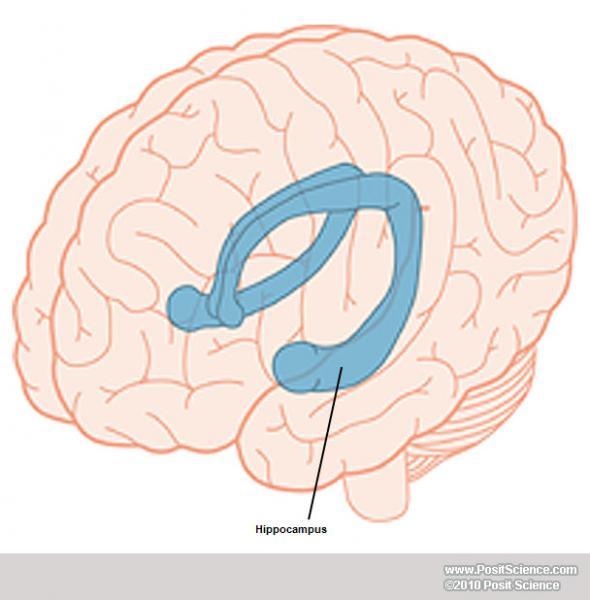
Furthermore, specimens from human organs are often sampled from a part of an organ, and it is generally not trivial to identify the exact original location and orientation of the analyzed sample. Spatial atlases for well-studied organs like the brain exist, but matching individual samples to a reference atlas is challenging as 2D sections may be angled and not well-aligned, and shape and size of organs differ between individuals. Highly multiplexed spatial expression analysis of genes is essential to uncover the organization of biological processes in relation to sub-regions of tissues and organs.
Mouse brain xsection manual#
We show that spatial gene expression data can be used for unsupervised and unbiased annotations of mouse brain spatial compartments based only on molecular markers, without the need of subjective manual annotations based on tissue and cell morphology or matching reference atlases. We also show that morphological variation does not always follow gene expression, and different spatial compartments can be defined by various cell types with common morphological features but distinct gene expression profiles. We apply our method on mouse brain sections and show that a fully unsupervised approach can computationally define anatomical compartments, which are highly reproducible across individuals, using as few as 18 gene markers. We also visualize gradients in gene expression and sharp as well as smooth transitions between different compartments. Here, we show how in situ sequencing data combined with dimensionality reduction and clustering can be used to identify spatial compartments that correspond to known anatomical compartments of the brain. With the advent of in situ sequencing technologies and automated approaches, it is now possible to profile the gene expression of targeted genes inside preserved tissue samples and thus spatially map biological processes across anatomical compartments. However, this task is challenging since sliced tissue sections are rarely perfectly parallel or angled with respect to sections in the reference atlas and organs from different individuals may vary in size and shape and requires manual annotation. Neuroanatomical compartments of the mouse brain are identified and outlined mainly based on manual annotations of samples using features related to tissue and cellular morphology, taking advantage of publicly available reference atlases.
